AI can hear you
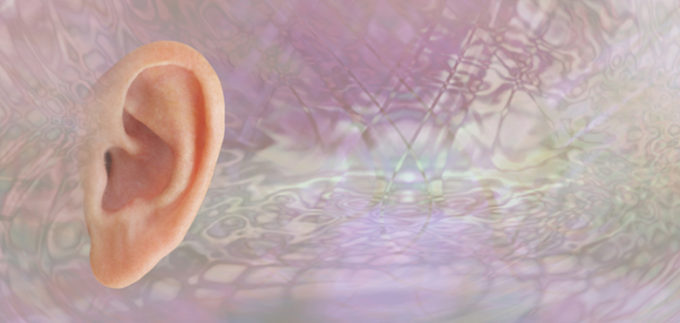
This post is written by Michiel Salters and Jasper van Dorp Schuitman from Sound Intelligence. Read more about Michiel and Jasper at the end of this post. |
Seeing and hearing go hand-in-hand when being aware of what is happening around us. So in security, it makes sense that audio as well as visual insight can help develop a better picture of what is actually occurring in the target area.
In this post, we hear from Michiel Salters, M.Sc., who is Technical Director and Jasper van Dorp Schuitman, PhD, Senior Scientist at Sound Intelligence about the importance of being able to identify and locate vital events in your security recordings using audio analytics.
Never miss an event
It’s impossible to be physically present everywhere at the same time. And like most people, we use video surveillance to see and hear what’s happening everywhere we are not. Easy enough with just a few cameras, but it’s not practical to physically monitor many cameras simultaneously. How long would it take to discover an important event on one camera while you are looking somewhere else? What would you miss? What would be the consequences?
This is why real-time edge-based analytics are so valuable – to detect and categorize events, and alert an operator to situations of interest. When you think about edge-based analytics on cameras, you probably think about video or image-based analytics, but they can also be audio analytics. For example, gunshots, aggression and breaking glass would be difficult to detect with image-based analytics, but can be quickly detected using audio analytics – even if the event is beyond the camera’s field of view. Early detection of these type of events means that security personnel or law enforcement can be dispatched to de-escalate a situation or reach victims quickly – potentially even save lives.
But how do audio analytics distinguish a gunshot from a slamming door? A group of loud teenagers from having fun or an argument? While early detection of a serious event is important, so too is minimizing the number of false alarms.
Better detection with machine learning
Audio and video analytics are two forms of Pattern Recognition, a branch of Artificial Intelligence (AI). AI has seen a revolution in the last decade, powered by Machine Learning. No longer is it necessary to painstakingly program all intelligence into an AI, instead one provides the AI with sample data and tells it to learn the patterns from that data. This idea is not new, but it only became feasible recently with the availability of affordable GPU’s. Originally developed for gaming, these chips turned out to be far more versatile than their developers envisaged. Key machine learning algorithms developed around the turn of the century suddenly became practical. Quite fortunately, these new techniques proved to be very flexible. Neural network algorithms for still image recognition could transfer to video and audio analytics as well.
However, the key to successfully applying these new techniques, is the dataset you have to work with. Training and testing machine learning models correctly requires datasets that are large and diverse enough to describe the variety and types of sounds you are interested in classifying. At Sound Intelligence, we have audio data from numerous real-life environments – data that’s been collected over the last twenty years and annotated manually in-house. The fact that we are able to apply cutting-edge machine learning on such a unique set of audio data makes us a leading company in the industry of real-life sound recognition.
Community-based innovation

The rapid development of AI was not just a matter of hardware and software. It also benefitted from an open community and close cooperation between academia and industry. AI tools are now freely available because large companies with big, in-house research departments, like Facebook and Google, recognize that collaboration speeds up development and benefits the whole community in the long run. In fact, a number of forums arrange AI competitions, where researchers are invited to test new ideas and algorithms on public data sets.
One such forum that we at Sound Intelligence have been involved with is DCASE (Detection and Classification of Acoustic Scenes and Events) – an annual series of AI challenges specific for audio analytics. Organized annually since 2016, it combines online challenges with a two-day workshop where the winners present their successful strategies. Hundreds of scientists from leading universities, research institutes and industry gather to discuss state-of-the-art technologies that can be used in future solutions.
Sound Intelligence co-sponsors this event together with companies like Amazon, Facebook, Google, IBM and Microsoft. The growing interest from these big names shows that the field of sound classification and detection is getting more and more attention. We also serve as industry experts at DCASE for reviewing and judging the challenge submissions, awarding those that are most relevant in our field.
The DCASE challenges are a great way to explore the boundaries of what is theoretically possible -with minimal limitations on processing power and time. The researchers working on the DCASE tasks typically have multiple GPUs at their disposal for running very complex algorithms; sometimes even multiple algorithms in parallel. However, in the real world, security applications have limited processing power and classifications need to happen in real-time. A big challenge for Sound Intelligence, and the AI community in general, is to apply state-of-the-art machine learning techniques in stand-alone devices for real-time applications.
Deploying cutting-edge analytics with Axis
For a practical deployment, analytics need to run on a more practical platform. An edge-based platform such as the AXIS Camera Application Platform (ACAP) is one such platform, transforming the camera into an intelligent device. Axis has also made great strides over the past years with introducing more processing power inside their network cameras and audio devices based on their ARTPEC chip. The newest ARTPEC-7 System-on-Chip with hardware support for Neural Networks makes machine learning-based acoustical analysis even more feasible.
With the increased amount of available processing power, both video and audio analytics can run in parallel. They can also be combined to yield even better detection quality, paving the way for future integration of audio and video meta-data and deep neural net training on the combined dataset.
Artificial intelligence – today and tomorrow
Artificial Intelligence is here to stay, and the technology matures every day. Open-sourced tools and data sets will make ‘basic’ AI accessible by everyone. Hardware innovation such as that in ARTPEC-7 will become commonplace, enabling even more complex AI. With the wide-spread availability of tools and hardware, the key differentiators for the next decade will not be who has the best AI components, but who best understands customer needs and who has the best quality data sets.
The Sound Intelligence deep neural networks are trained on real-world environments and as a result, work in real-world environments. Working closely with Axis to continually improve our respective hardware and software solutions, we are meeting customer needs across a variety of industry segments and environments.
![]() Michiel Salters, M.Sc. is Technical Director at Sound Intelligence. A graduate of the Pattern Recognition group at Delft University of Technology, he previously worked at CMG consultancy and TomTom. He’s been finding patterns in telephony traffic, traffic jams and now in audio. |
![]() Jasper van Dorp Schuitman, PhD is Senior Scientist at Sound Intelligence. He received his PhD in applied physics at Delft University of Technology and performed research in the fields of audio reproduction and recording, room acoustics, modeling of the human auditory system, audio watermarking and fingerprinting and sound event detection. |
June 23, 2020 9:06 pm|
Like!! I blog quite often and I genuinely thank you for your information. The article has truly peaked my interest.